From seemingly out of nowhere, artificial intelligence (AI) and two of its subsets, machine learning (ML) and deep learning (DL), have emerged as powerful forces driving innovation across various industries. As for AI for GIS (Geographic Information Systems), AI, ML, and DL have unlocked new possibilities, propelling the field into a new era of geospatial analysis. For innovative companies already utilizing GIS applications and high-resolution aerial orthoimagery, these cutting-edge technologies promise to revolutionize the way geospatial data is analyzed and put to work.
What is Artificial Intelligence in GIS?
Artificial Intelligence refers to the broader discipline of creating machines or systems that exhibit human-like intelligence. AI encompasses a wide range of techniques, methods, and approaches to enable machines to simulate human intelligence and perform tasks that typically require human cognitive abilities.
Artificial intelligence (AI) in GIS refers to the integration of machine learning techniques into Geographic Information Systems to enhance data analysis, interpretation, and decision-making processes.
The Rise of Machine Learning & Deep Learning
When analyzing geospatial data, GIS and artificial intelligence are a natural fit. Two subsets take center stage: machine learning and deep learning. Each has different approaches and applications. Machine learning provides a broader range of statistical techniques, while deep learning excels at image analysis and complex pattern recognition tasks.
Here’s a breakdown of their differences and examples of how they are used in GIS geospatial applications:
Machine Learning:
Machine learning involves the development of algorithms that can learn from data and make predictions or decisions without being explicitly programmed. It focuses on using statistical techniques to enable computers to learn patterns and relationships in data.
Examples in GIS Geospatial Applications:
- Classification: Machine learning algorithms can be used to classify land cover types in aerial imagery, such as differentiating between forests, agricultural fields, and urban areas.
- Regression: Machine learning models can predict property values based on various spatial attributes like location, proximity to amenities, and infrastructure.
- Clustering: Machine learning algorithms can group similar geographic features together, such as clustering similar points of interest or identifying similar weather patterns.
Deep Learning:
Deep learning is a subset of machine learning that mimics the structure and functionality of the human brain’s neural networks. It involves training deep neural networks with multiple layers to automatically learn hierarchical representations of data.
Examples in GIS Geospatial Applications:
- Object Detection: Deep learning models can identify and detect specific objects, such as buildings, roads, and vehicles, in aerial imagery.
- Image Segmentation: Deep learning algorithms can segment an image into different regions or objects, allowing for more detailed analysis. For example, extracting individual trees from LiDAR data to estimate forest canopy density.
- Natural Language Processing: Deep learning techniques can extract geospatial information from unstructured text data, such as extracting addresses or points of interest from social media posts or online reviews.
Prius Intelli is proud to be part of the Esri Partner Network. Explore the deep learning toolsets currently available in ArcGIS Pro.
Putting AI in GIS to Work for You
With AI and machine learning at their disposal, industries can unlock untapped potential and gain a competitive edge in their respective domains. Here are five key advantages businesses enjoy when putting AI in GIS to work across their operations.
- Improved Data Analysis: With machine learning, vast amounts of geospatial data can be processed more efficiently than traditional methods. By automatically recognizing patterns and relationships within the data, machine learning and deep learning models can uncover insights and trends that humans might miss. This leads to more accurate and comprehensive data analysis in GIS applications.
- Enhanced Spatial Analysis: Machine learning algorithms can be trained to recognize spatial patterns and relationships, allowing for advanced spatial analysis. For example, clustering algorithms can identify groups or clusters of similar geographic features, such as land cover types or population densities. This enables better understanding and visualization of spatial phenomena, supporting effective decision-making.
- Precise Prediction and Forecasting: Spatial regression and time series analysis techniques can be leveraged to make accurate predictions and forecasts in GIS applications. For instance, machine learning models can predict future land use changes, population growth, or environmental conditions based on historical data. This information is valuable for pipeline route planning, urban planning, risk assessment, and resource management.
- Automated Feature Extraction: Machine learning algorithms can automate the process of feature extraction from remote sensing data. For example, deep learning models can identify and classify objects like buildings, roads, vegetation, and water bodies from aerial imagery. This image classification saves time and effort compared to manual feature extraction, enabling faster updates to GIS databases.
- Real-time Decision Support: Machine learning algorithms can process data in real-time, allowing for dynamic decision-making in GIS applications. For example, predictive models can analyze incoming data streams to detect anomalies, such as natural disasters or disease outbreaks. This enables timely response and mitigation strategies to be implemented, helping to save lives and minimize damages.
Intelligent Technologies Offer a Boundless Frontier of Innovation
The integration of AI and machine learning in industries has proven to be a game-changer in data analysis, spatial analysis, prediction and forecasting, and automated feature extraction. The ability to process large quantities of geospatial data efficiently, uncover valuable insights, make accurate predictions, and automate time-consuming tasks has revolutionized how industries operate. With these advancements, industries can make more informed decisions, optimize processes, and gain a competitive edge in today’s rapidly evolving landscape. As AI continues to advance, the potential for further innovation and transformation across industries is boundless, driving us towards a future where intelligent technologies play a central role in shaping our world.
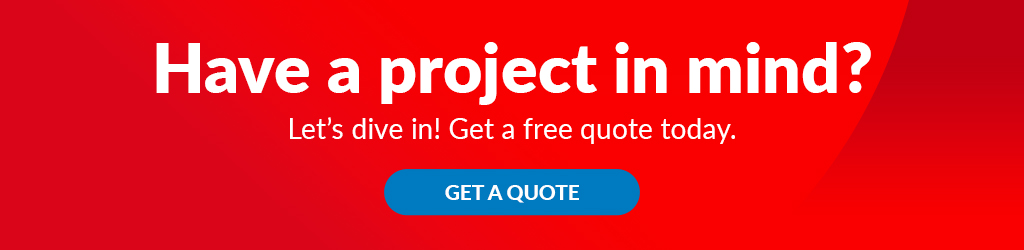
Related Information
- How Geospatial Data Modeling Sheds Light on Reality
- Exploring Geospatial Data Solutions: Mapping the World with Precision
How Up to Date is Google Earth? Do You Really Know?